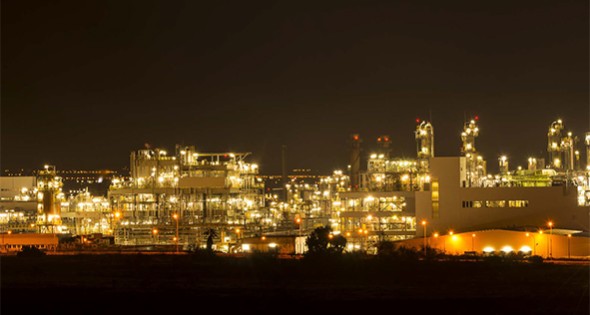
The Industrial Manufacturing Revolution Is Already Under Way
19 Jul, 2019
The digitization of industrial manufacturing continues to move the needle in advancing how the industry grows and what that means to the bottom line. Dramatic changes are under way, and not all industrial manufacturers are able to make it work for their business.
According to the PricewaterhouseCoopers (PwC) report “Industrial Manufacturing Trends 2018-2019,” a significant portion of new sales growth for industrial equipment manufacturers in the immediate future will come from connected equipment with sensors, actuators, and analytical insights that can exchange critical data with other machines and computer networks in real time via the cloud. “Data shows that across all industries, companies that earmark money for research and development spending on software earlier than their competitors enjoy greater revenue gains,” the report stated.
The report also stated that 72 percent of manufacturing companies surveyed by PwC said they are dramatically increasing their level of digitization and expect to be able to be ranked as digitally advanced by 2020, compared with just 33 percent today. These companies are committing $907 billion per year — about 5 percent of revenues — toward greater connectivity and smarter factories.
Right now, digitally-advanced industrial manufacturers either are collaborating with other companies to develop new digital technologies that they can own and embed into their products in a unique way, or they are creating technologically focused research units that operate almost as separate companies, according to the PwC report.
Collaboration Examples
A good example of the collaboration approach is the effort between Schindler Group, which makes elevators, escalators, and other mobility equipment, and General Electric. Schindler will leverage GE’s Predix Platform and GE’s proven digital industrial expertise in advanced analytics to further its predictive analytics and machine learning capabilities. With Predix, Schindler will enhance its capabilities to monitor, analyze, and leverage data generated from its connected elevators and escalators, and will enable Schindler to predictively identify, analyze, and resolve possible service issues before they happen, reducing and eliminating downtime.
IBM recently announced a new portfolio of Internet of Things (IoT) solutions that team artificial intelligence (AI) and advanced analytics to help asset-intensive organizations, such as the Metropolitan Atlanta Rapid Transit Authority (MARTA), improve maintenance strategies.
The solution is designed to help organizations lower costs and reduce the risk of failure from physical assets such as vehicles, manufacturing robots, turbines, mining equipment, elevators, and electrical transformers.
IBM Maximo Asset Performance Management (APM) solutions collect data from physical assets in near real-time and provide insights on current operating conditions, predict potential issues, identify problems, and offer repair recommendations.
According to an IBM press release, business leaders can now know “if a machine is likely to fail, using advanced analytics and AI to understand timing, predict consequences, and identify problems.”
It’s all part of IBM’s “asset health insights” designed to provide asset health assessments in near real-time using asset records, sensor data, and other external data to inform maintenance and replacement decisions; use predictive maintenance insights to predict asset health using statistical models and machine learning that includes failure date/probability, key drivers, degradation curves, and anomaly detection; and offer equipment management assistance that enables technicians to repair equipment with an AI-powered assistant providing quicker access to documentation, diagnostics and recommendations for repair.
IBM will also offer the APM suite customized for specific industries, beginning with APM for Energy and Utilities (E&U). This provides industry-specific capabilities to analyze and act on insights from utility assets and includes risk/criticality scoring, health and degradation models, standard industry data model, and weather data integration.
The New Evolution of Industrial Manufacturing
The Industrial Internet Consortium, a membership program transforming business and society by accelerating the industrial IoT, calls this new evolution of industrial manufacturing the “symphonies of systems” that facilitate real-time interactions between machines, assets, systems, and things. “This is the smart factory, the factory of the future…permitting processes to govern themselves, with intelligent machines and devices that take corrective action to avoid unscheduled breakdowns. Downtime becomes a thing of the past, waste and defects eliminated; each machine moves in perfect time. Every handheld digital device in the factory reports the status of every fixed device, giving personnel access to real-time, actionable information. Wearable technology tracks employee location in case of emergency.”
The consortium envisions a future where customization will be automatic; raw materials will be programmed with information that it will be part of product X, to be delivered to customer Y; once the material is in the factory, the material itself records any deviations from the standard process, determines when it’s “done,” and knows how to get it to its customer.
Bobby Bono, industrial manufacturing leader for PricewaterhouseCoopers, wrote in 2014 about challenges to industrial manufacturing in Industry Today magazine, including results being impacted by volatile currency movements; emerging markets experiencing slowdowns in sectors such as mining, infrastructure, etc.; declining commodity prices that are starting to limit some of the investments; cautious consumer spending causing slowdowns in some industries (e.g., electronics); demand that is expected to remain muted in the near term; and customers taking longer to make decisions on new orders.
The real focus on technological change in industrial manufacturing is more about getting closer to the customer, he told BXJ. “I think you are seeing now there are two ways industrial companies are trying to innovate,” Bono says. “Inside their four walls, it’s about how they do manufacturing and make it more efficient in their operations. But there is also the thought process of how they can innovate in their products that they are selling to their customers.”
It’s all about using sensors to gather data and collect predictive analytics to make the manufacturing process more efficient. “It’s also how can I insert sensors into my products to monitor the efficiency of my product in the real world to gather data so that I know where there is going to be downtime and when it’s about to break, so I can send someone out there to anticipate that and fix that problem before it breaks,” he says. “That way, my customer doesn’t have problems. But that real-time monitoring, and that predictive analytics, is also a new revenue stream that I can sell to my customer.”
Bono sees a future that goes even further in getting closer to the customer. For instance, a customer has a washing machine with built-in monitoring. “We are not quite there yet, but we can get to a point where the manufacturer will send you the parts that you need to fix your washing machine, and you use 3D goggles in an augmented reality scenario to see how to fix it. Then you can fix it yourself.”
One example of how analytics works in industrial manufacturing comes from a case study cited by EFT Analytics, a subsidiary of Koch Industries that provides software-as-a-service (SaaS). For one of the world’s most technologically advanced steel mills, Big River Steel, in Arkansas, EFT’s advanced analytics software platform and engineering expertise was combined with Big River Steel’s process and steel-making knowledge. EFT delivered a real-time solution to predict product quality issues and provide operators with guidance for reducing defects before they occur. “We view data analytics and machine learning as game-changing solutions that allow us to identify and avoid the root causes of defects,” Dave Stickler, CEO of Big River Steel, said in a press release.
Another case study from EFT discussed a printing plant that experienced difficulties with a machine that would frequently break when pushing additional throughput. The number of transfer breaks varied daily and were purportedly random in nature. A transfer break is categorized as the failure of the undried sheet to “transfer” from the felt onto the backing roll. This resulted in the sheet breaking and downtime being incurred to rethread it onto the machine. Running this machine reliably would mitigate a loss of $1 million per month.
EFT initially utilized over 4,500 variables provided for analysis. Through evaluation, these variable lists were reduced to 600. A predictive model was built with the client’s process knowledge experts in order to determine the probability of these transfer breaks. With the amount of reduced variable counts and use of correlations, EFT and the client were able to find a weekly anomaly causing a significant amount of these breaks.
Through this effort, the client’s subject matter experts were able to identify a relationship using four variables, ultimately saving them $12 million annually in wasted production.
What To Do Now
Another PwC report outlined what industrial manufacturers should be doing now to create a nimble, new workplace: start attracting talent now, and be more open to where they will find their best employees; invest in education and training, because in-house training as well as external partnerships will help to prepare the existing employee base to program, operate and maintain the robots and digitally enabled machinery; and remake the workplace culture, because they now must compete with tech companies and startups to attract millennial talent.
Bono says he is seeing some of that movement now. “I am seeing manufacturers trying to rebrand themselves as tech companies to try to retain those millennial employees. You need somebody now that can capture data, interpret that data, and then figure out what to do as a result of what that data is telling them,” Bono says. “The skill-set that manufacturing needs is a critical thing, and is hampering some of their development to adopt. The trade schools need to train them in a different way.”
Related Posts
-
Agri-technology adoption begins in earnest amidst the digitization of farming
-
New Sustainability, New Power Handling Ideas are Part of Data Center Evolution
-
A Huge Industry Begins Reworking Itself
-
Airports Signaling There’s a Recovery on the Horizon
-
Global and U.S. Seaports Struggling in a State of Economic Flux
-
More Foreign Trade Zones Handling an Ever Growing Bounty of Goods
-
Business Services and Strategic Planning
-
Innovations Driving Demand
-
Intermodal Expansion
-
Development with Wings